Bad Likert judge: the phrase itself conjures images of survey chaos, doesn’t it? We’re talking about those respondents who throw a wrench into your meticulously crafted research, skewing your data with inconsistent, biased answers. This isn’t just about a few stray marks on a form; it’s about understanding the subtle (and not-so-subtle) ways people can undermine the integrity of your Likert scale data. Get ready to dive into the world of response biases, inconsistent patterns, and how to salvage your research from the clutches of the “bad Likert judge.”
This deep dive explores the various facets of unreliable Likert scale responses, from identifying the telltale signs of a “bad Likert judge” to employing strategies to mitigate bias and improve data quality. We’ll dissect common response biases, analyze response patterns, and provide practical tips for designing better surveys and handling problematic data. We’ll even showcase real-world examples (hypothetical, of course!) to illustrate just how much a single unreliable respondent can impact your findings.
Defining “Bad Likert Judge”
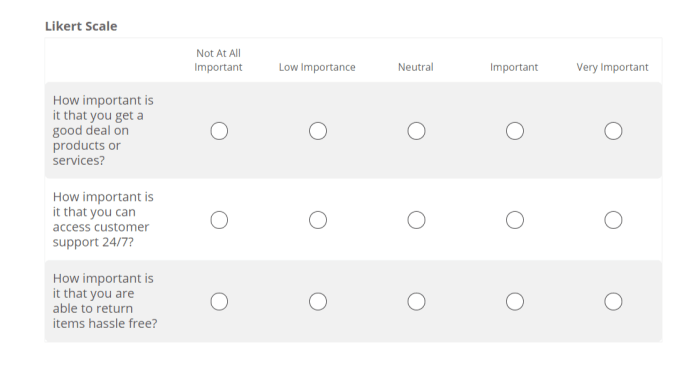
Source: wpforms.com
Understanding the concept of a “bad Likert judge” requires a nuanced perspective. It’s not about a person’s inherent character, but rather their approach to responding to Likert scale questions. A bad Likert judge provides responses that are unreliable, inconsistent, or systematically biased, ultimately compromising the validity of the data collected. This unreliability stems from a variety of factors, making it crucial to understand the different ways a respondent can skew results.
A reliable Likert scale response reflects a true and consistent internal state. In contrast, a bad Likert judge’s responses are characterized by inconsistency, a tendency towards extreme ratings, or a systematic bias towards certain response options, irrespective of the actual question. This can manifest as response set bias, where individuals consistently select the same response option regardless of content, or acquiescence bias, where they agree with statements regardless of their actual opinion. The result is data that doesn’t accurately represent the underlying attitudes or beliefs being measured.
Characteristics of a Bad Likert Judge
Several characteristics distinguish a bad Likert judge from a reliable one. These individuals may exhibit a tendency towards extreme responses (always choosing “strongly agree” or “strongly disagree”), demonstrating a lack of nuanced judgment. They might also display response set bias, consistently choosing the middle option (“neutral”) or another specific option regardless of question content. This indicates a lack of engagement with the questions and a failure to provide thoughtful answers. Further, a bad Likert judge might demonstrate acquiescence bias, consistently agreeing with statements regardless of their actual content, suggesting a lack of critical evaluation. Finally, they may show social desirability bias, answering in a way they perceive as socially acceptable, rather than truthfully.
Types of Biases Affecting Likert Scale Responses
Different types of biases significantly impact the reliability of Likert scale responses. Response set bias, as mentioned, refers to the tendency to consistently choose the same response option regardless of the question’s content. This can lead to artificially inflated or deflated scores. Acquiescence bias, or yea-saying, is the tendency to agree with statements regardless of their content. This is often driven by a desire to please the researcher or avoid appearing disagreeable. Conversely, nay-saying is the opposite, where respondents consistently disagree. Social desirability bias involves responding in a way that presents oneself in a favorable light, potentially distorting the true attitudes or beliefs. Finally, extremity bias reflects a tendency to choose extreme response options (strongly agree/strongly disagree), neglecting the more nuanced middle ground.
Hypothetical Scenario Illustrating Impact
Imagine a study assessing customer satisfaction with a new product. A significant portion of respondents consistently select “strongly agree” to every question, regardless of the question’s positive or negative framing. This represents a clear case of response set bias or acquiescence bias. The resulting data would inflate the perceived customer satisfaction, leading to inaccurate conclusions about the product’s success. The researchers might invest further resources based on this flawed data, ultimately leading to a misallocation of resources and potentially impacting the product’s development. This illustrates how a group of “bad Likert judges” can significantly skew research findings and lead to incorrect interpretations.
Identifying Sources of Bias in Likert Responses
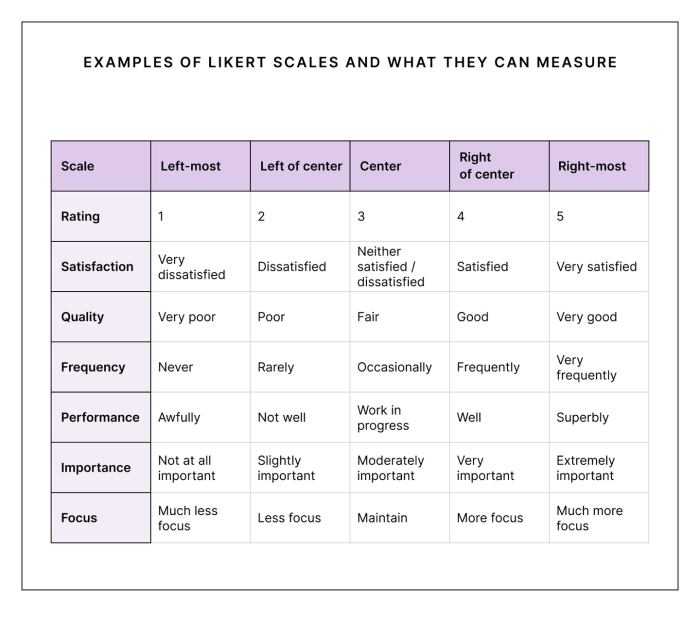
Source: cultureamp-cdn.com
Likert scales, while seemingly straightforward, are susceptible to various biases that can significantly skew results and undermine the validity of research findings. Understanding these biases is crucial for researchers aiming to collect accurate and reliable data. Ignoring these pitfalls can lead to flawed interpretations and ultimately, incorrect conclusions. This section delves into the common sources of bias in Likert responses, exploring their methodological challenges and offering strategies for mitigation.
Several response biases frequently contaminate Likert scale data. These biases stem from the respondent’s tendencies, rather than their true attitudes or beliefs. Understanding these tendencies is the first step toward improving data quality.
Common Response Biases in Likert Scales
Acquiescence bias, also known as “yea-saying,” refers to the tendency of respondents to agree with statements regardless of their content. This is particularly problematic in surveys with many positively worded items. Conversely, extremity bias reflects a respondent’s preference for selecting the most extreme response options, avoiding the middle ground. This can lead to an artificial inflation or deflation of scores, depending on the nature of the items. Central tendency bias, on the other hand, represents the opposite – respondents consistently choosing the neutral or middle option, potentially masking their true opinions. Finally, social desirability bias occurs when participants answer in a way they believe will be viewed favorably by others, potentially distorting their genuine responses.
Methodological Challenges in Mitigating Bias
Mitigating response biases in Likert scales presents significant methodological challenges. One major hurdle is the inherent subjectivity involved in both the design of the questionnaire and the interpretation of the responses. Researchers must carefully craft questions to avoid ambiguity and leading phrasing. However, even with meticulous design, the respondent’s individual characteristics and situational factors can still influence their responses. The lack of a universally accepted “best” method for bias reduction further complicates matters, requiring researchers to carefully consider the specific context of their study when choosing an approach.
Influence of Participant Characteristics on Responses
Participant characteristics such as age, education, and cultural background can significantly influence Likert scale responses. For example, older adults might exhibit a greater tendency toward acquiescence bias compared to younger participants. Similarly, individuals with higher levels of education may be more inclined to express nuanced opinions, potentially leading to a wider distribution of responses across the scale, compared to those with lower education levels. Cultural norms also play a role; in some cultures, expressing extreme opinions might be considered socially unacceptable, leading to a greater prevalence of central tendency bias. Consider a study on job satisfaction: older workers, potentially more accustomed to accepting their work situations, might show lower scores than younger workers who might be more likely to express dissatisfaction.
Bias Mitigation Techniques
Technique | Strengths | Weaknesses | Example |
---|---|---|---|
Balanced Scales (equal number of positive and negative items) | Reduces acquiescence bias | Can be more complex to design and analyze | Including both “I am satisfied with my job” and “I am dissatisfied with my job” |
Reverse-scored items | Helps detect acquiescence bias | Can be confusing for respondents | Including an item like “I am *not* satisfied with my job” |
Item wording variation | Reduces bias from specific phrasing | Requires more items | Rephrasing items to avoid leading language |
Ensuring anonymity | Reduces social desirability bias | May not eliminate it completely | Using anonymous online surveys |
Using a forced-choice format | Reduces central tendency bias | Can lead to less nuanced responses | Presenting two options and requiring a choice between them. |
Analyzing Response Patterns
Unmasking a “bad Likert judge” isn’t about finding the *worst* responses, but identifying patterns that suggest unreliability. Think of it like detective work – you’re looking for inconsistencies that hint at a respondent not engaging thoughtfully with the survey. These patterns, when properly identified, can significantly improve the quality of your data analysis.
Analyzing response patterns helps us weed out data that might skew results. We’re not interested in judging respondents, but in ensuring the data accurately reflects the opinions being measured. This involves looking for both obvious and subtle signs of inconsistent or erratic responses. By understanding these patterns, we can confidently rely on the remaining data for meaningful insights.
Identifying Inconsistent Responses
Detecting inconsistent responses requires a systematic approach. One common method is to examine response frequencies for each item. A respondent consistently choosing the “neutral” option across all items, for instance, suggests a lack of engagement or a tendency towards response bias. Similarly, a respondent who alternates sharply between extreme ends of the scale (“strongly agree” and “strongly disagree”) without apparent reason warrants further investigation. This inconsistency could stem from carelessness, misunderstanding of the questions, or a deliberate attempt to sabotage the survey. Software packages often provide tools to easily visualize response distributions, helping identify such anomalies. For example, a histogram showing a highly skewed distribution for a specific item compared to others could signal a problem.
Detecting Erratic Responses Across Items
Beyond individual item analysis, we need to examine the overall response pattern across multiple items. Imagine a survey measuring attitudes toward environmental protection. A respondent who strongly agrees with statements promoting conservation but strongly disagrees with statements supporting environmental regulations displays an internal inconsistency. This discrepancy suggests a lack of coherent thought or perhaps a misunderstanding of the nuances within the questions. To detect this, we can calculate correlation coefficients between items. Low or negative correlations between items that should logically be related hint at erratic responding. For example, two items asking about support for recycling programs should show a strong positive correlation. A low or negative correlation would raise a red flag.
A Step-by-Step Process for Analyzing Response Patterns (Flowchart Description)
Imagine a flowchart. The first step would be data import and cleaning. Then, we’d calculate descriptive statistics for each item (mean, standard deviation, frequency distribution). Next, we’d visualize these distributions (histograms, box plots) to identify potential outliers or unusual patterns. Following this, we would compute inter-item correlations to detect inconsistencies across related items. Finally, we’d apply statistical techniques (e.g., Mahalanobis distance) to identify outliers, which are respondents whose response patterns deviate significantly from the majority. Respondents flagged as outliers would then be reviewed individually to assess the validity of their responses. If necessary, those responses could be removed from further analysis.
Statistical Techniques for Identifying Outliers
Several statistical methods help pinpoint unreliable respondents. One powerful technique is the Mahalanobis distance. This measures the distance of a respondent’s response vector from the centroid (mean) of all response vectors, accounting for the correlation between items. Respondents with exceptionally high Mahalanobis distances are considered outliers. Another method involves using factor analysis to identify latent constructs and then examining the factor scores for each respondent. Extreme factor scores could signal inconsistencies in their responses. These statistical techniques provide objective measures to identify potentially problematic responses, allowing for a data-driven approach to cleaning the dataset. The key is to use these methods judiciously, considering the context of the survey and the potential reasons for outliers. Simply removing all outliers is not always the best approach; careful examination of each case is crucial.
Improving Likert Scale Data Quality
So, you’ve got your Likert scale data, but it’s looking a little… messy. Don’t worry, we’ve all been there. The good news is that you can take steps to improve the quality of your data, leading to more reliable and insightful results. Let’s dive into some practical strategies to boost your Likert game.
Improving the quality of your Likert scale data hinges on careful planning and execution. From the initial design to the final data analysis, attention to detail can dramatically reduce bias and increase the validity of your findings. Think of it as crafting the perfect recipe – each ingredient (step) plays a crucial role in the final product (reliable data).
Likert Scale Design Enhancements for Bias Reduction
Minimizing bias in Likert scales starts with smart design choices. Avoid leading questions that subtly nudge respondents towards a particular answer. For instance, instead of “Do you strongly agree that our product is amazing?”, try a more neutral phrasing like “How would you rate our product’s overall quality?”. Keep response options balanced and clearly defined. Using an even number of options (e.g., 4 or 6 points) can force respondents to make a more considered choice, as opposed to a neutral midpoint. Consider also using visual aids, such as visual analogue scales (VAS), which can be particularly useful when dealing with subjective experiences like pain or satisfaction. A VAS might be a 10cm line, anchored at each end with labels such as ‘no pain’ and ‘worst imaginable pain’, allowing participants to mark their level of pain along the line. This provides more nuanced data than simple categorical responses.
Best Practices for Respondent Instruction
Clear and concise instructions are key. Explain the purpose of the scale, what each response option means, and how respondents should select their answers. Provide examples to illustrate how to use the scale, particularly if it is complex or involves multiple dimensions. For online surveys, ensure the layout is intuitive and easy to navigate, with clear visual cues to guide respondents through the process. Consider using a pilot test with a small group to identify any potential ambiguities or difficulties in understanding the instructions.
Pre-testing Likert Scales: A Crucial Step
Pre-testing, also known as pilot testing, is like a dress rehearsal before the main event. It allows you to identify potential problems with your scale before it’s deployed to a larger sample. Conduct a small-scale test with a group similar to your target population. This helps identify confusing questions, ambiguous response options, and any issues with the overall survey design. Analyzing the pilot data allows you to refine your scale and ensure it is ready for prime time. It might reveal that a particular question is consistently misinterpreted or that certain response options are rarely selected, signaling a potential problem with your scale’s design.
Strategies for Handling Missing Data and Inconsistent Responses
Missing data and inconsistent responses are inevitable. However, you can minimize their impact. For missing data, explore different imputation techniques. Simple methods include replacing missing values with the mean or median of the available data. However, more sophisticated techniques, like multiple imputation, are available and can provide more robust results, particularly for larger datasets. Inconsistent responses, such as those that show a pattern of selecting only extreme options, might suggest response bias. These responses should be carefully considered and may require exclusion from the analysis, or at least flagged for further investigation.
Illustrative Examples of Bad Likert Judge Behavior
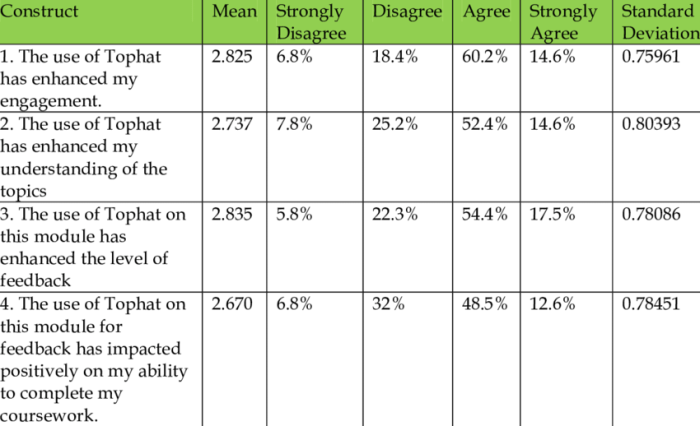
Source: researchgate.net
Understanding how respondents can skew Likert scale data requires looking at real-world scenarios. Let’s examine some hypothetical examples of “bad Likert judging” to highlight the pitfalls and their consequences. These examples illustrate how seemingly minor variations in response patterns can significantly impact the reliability and validity of research findings.
Example 1: The “Extreme Responder”
Imagine a survey on customer satisfaction with a new phone. Sarah, a participant, consistently selects either “strongly agree” or “strongly disagree” for every question, regardless of the actual sentiment. This behavior, known as extreme responding, introduces bias by artificially inflating the distribution at the ends of the scale. Sarah’s responses show a complete lack of nuance, failing to reflect the subtleties of her experience. Including such responses in data analysis would distort the overall customer satisfaction score, potentially leading to inaccurate conclusions about the product’s strengths and weaknesses. For instance, if a majority of respondents are more neutral, Sarah’s responses would skew the mean towards the extreme ends, making the results unreliable.
Example 2: The “Acquiescent Responder”
John participates in a survey about workplace morale. He consistently agrees with every statement, regardless of its wording or content. This acquiescence bias, or yea-saying, distorts the data by artificially inflating agreement across the board. For example, he might agree with both “I am satisfied with my current workload” and “My workload is overwhelming.” The consequence of including John’s responses is a skewed perception of positive morale, even if underlying issues exist. The true sentiment of the workforce remains obscured, potentially leading to flawed management decisions based on misleadingly positive data.
Example 3: The “Midpoint Responder”
Maria takes a survey on political opinions. She consistently chooses the neutral midpoint (“neither agree nor disagree”) for every question, avoiding expressing a clear preference. This midpoint responding, or neutral responding, introduces bias by reducing the variance in the data and obscuring genuine opinions. It’s difficult to extract meaningful information from Maria’s responses, as they provide no indication of her actual political leanings. Including these responses in the analysis would dampen the overall distribution, making it harder to identify meaningful trends or correlations within the political preferences of the surveyed population. The result is a diluted and less informative dataset.
Visual Representation of Bad Likert Judge Impact
Imagine a histogram representing the distribution of responses to a Likert scale question. A normal distribution would show a bell curve, with most responses clustered around the mean. However, the presence of a “bad Likert judge” – say, an extreme responder – would dramatically alter this picture. The histogram would show a significant spike at one end of the scale (“strongly agree” or “strongly disagree”), while the rest of the distribution would appear flattened or skewed. This visually represents how one unreliable respondent can significantly distort the overall data distribution, making it harder to draw accurate conclusions. The mean and standard deviation would be significantly impacted, rendering any statistical analysis based on this distorted data questionable. The bell curve would be severely distorted, with a long tail extending towards the extreme end, reflecting the disproportionate influence of the “bad Likert judge.”
Final Summary: Bad Likert Judge
So, you’ve learned to spot a “bad Likert judge” from a mile away. You’re armed with the knowledge to identify response biases, analyze response patterns, and implement strategies to improve the quality of your Likert scale data. Remember, it’s not about eliminating every slightly off-kilter response; it’s about understanding the nuances of human behavior and employing the right techniques to ensure your research remains reliable and meaningful. The quest for data integrity is ongoing, but with the right tools and understanding, you can navigate the tricky world of Likert scales with confidence.